The price of a bit and the tear tracks of the cheetah
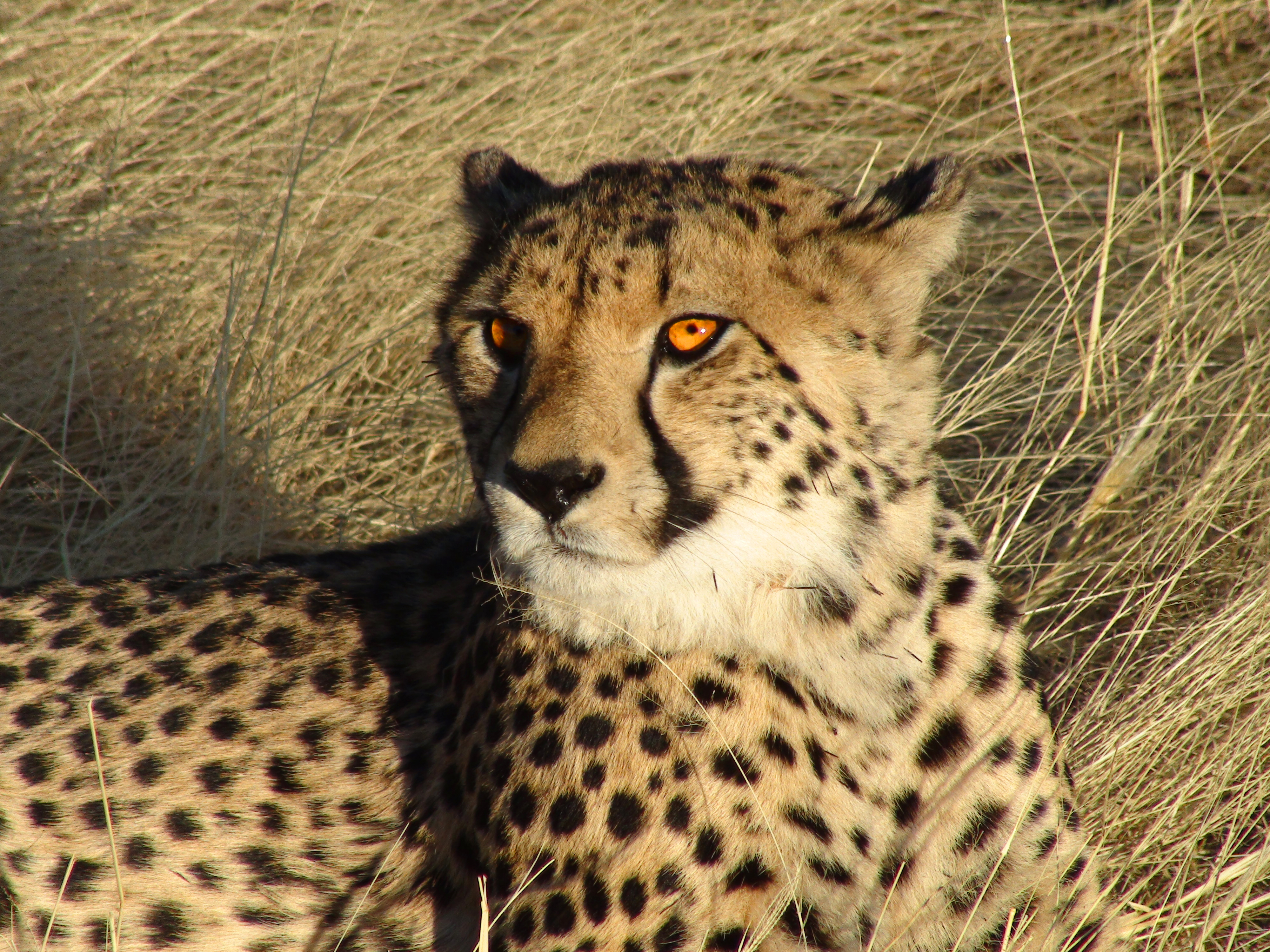
Barath Raghavan and Bruce Schneier have written a piece proposing an AI dividend. I like the idea, and I’d like to riff on their pricing strategy a bit.
The unreasonable effectiveness of some people’s data
Their model focuses on the use of public data, which belongs equally to every member of the public, rather than to a smaller group of rights-holders, and the authors make it clear that this system is not intended to replace or interfere with copyright protections. But I suspect that copyright-protected data is part of a class of training data that raises interesting questions about data pricing: the Unreasonably Effective class.
🚿 While the bulk of the data used to train AI may indeed be public, I wonder if there might be a Pareto principle operating, where some smaller proportion of the data provides much of the model’s utility. For example, assume good data hygiene and good data citizenship could create an AI about 80% as useful as GPT3.5 – that would not be useful enough to be commercially viable.
Given current usage patterns, a large amount of utility must derive from OpenAI’s ingestion of grey-area material like Stack Overflow, Quora, and recipe sites like Epicurious.
This marginal utility problem is probably most visible in the image generation space. Here pixel quality really matters: terabytes of low-res museum scans uploaded years ago, and amateur drawings on Flickr and Pinterest probably don’t yield the same results as the 10% of the data that consists of well-tagged high-resolution digital art portfolios from masters like Greg Rutkowski. The first generation of production-grade generators essentially told on itself by using “$famous_digital_artist_name” as a prompt sweetener to create higher-quality images.
Here we have the 80/20 problem, visualized.
Paying in tranches
So when I return to the question of an appropriate AI dividend, I think the distinction between the bulk data used to scaffold and and fill the model and the high-marginal-utility data used to give it fine detail is important. It seems fair that these parts of the dataset should be compensated at a higher rate.
Thinking about how this principle might be operationalized brings up the problem of differential data valuation in general. My intuition is to treat it as an information theory problem, where the value of each bit is its contribution to resolving the signal.
For example, for people not deeply familiar with the coat patterns of the larger cats, it maybe difficult to distinguish a drawing of a leopard from a drawing of a cheetah – until you look at the facial mask, and see the black marks leading from the eyes to the muzzle. Cheetah it is.
So the question becomes: how do you identify the tear tracks of a ChatGPT recipe? Of a D&D character portrait?
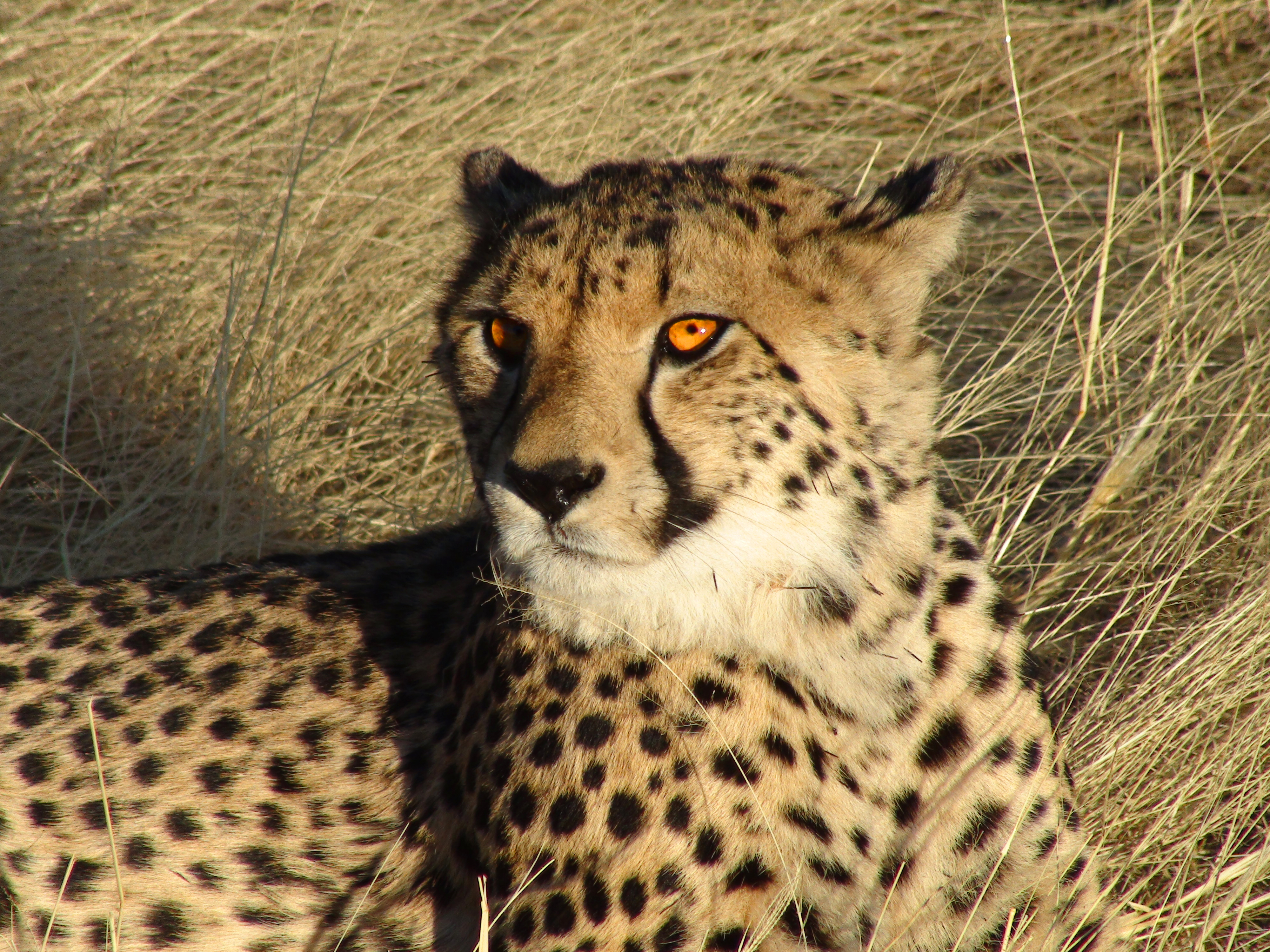